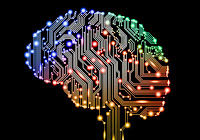
extending my previous article about "Using AWS Machine Learning from ABAP to predict runtimes" I have now been able to extend the ABAP based API to create models from ABAP internal tables (which is like a collection of records, for the Non-ABAPers ;-).
This basically enables ABAP developers to utilize Machine Learning full cycle without ever having to leave their home turf or worry about the specifics of the AWS Machine Learning implementations.
My use case still is the same: Predicting runtimes of the SNP System Scan based on well known parameters like database vendor (e.g. Oracle, MaxDB), database size, SNP System Scan version and others. But since my first model was not quite meeting my expectations I wanted to be able to play around easily, adding and removing attributes from the model with a nice ABAP centric workflow. This probably makes it most effective for other ABAP developers to utilize Machine Learning. So let's take a look at the basic structure of the example program:
1: REPORT /snp/aws01_ml_create_model.
2:
3: START-OF-SELECTION.
4: PERFORM main.
5:
6: FORM main.
7: *"--- DATA DEFINITION -------------------------------------------------
8: DATA: lr_scan_data TYPE REF TO data.
9: DATA: lr_prepared_data TYPE REF TO data.
10: DATA: lr_ml TYPE REF TO /snp/aws00_cl_ml.
11: DATA: lv_model_id TYPE string.
12: DATA: lr_ex TYPE REF TO cx_root.
13: DATA: lv_msg TYPE string.
14:
15: FIELD-SYMBOLS: <lt_data> TYPE table.
16:
17: *"--- PROCESSING LOGIC ------------------------------------------------
18: TRY.
19: "fetch the data into an internal table
20: PERFORM get_system_scan_data CHANGING lr_scan_data.
21: ASSIGN lr_scan_data->* TO <lt_data>.
22:
23: "prepare data (e.g. convert, select features)
24: PERFORM prepare_data USING <lt_data> CHANGING lr_prepared_data.
25: ASSIGN lr_prepared_data->* TO <lt_data>.
26:
27: "create a model
28: CREATE OBJECT lr_ml.
29: PERFORM create_model USING lr_ml <lt_data> CHANGING lv_model_id.
30:
31: "check if...
32: IF lr_ml->is_ready( lv_model_id ) = abap_true.
33:
34: "...creation was successful
35: lv_msg = /snp/cn00_cl_string_utils=>text( iv_text = 'Model &1 is ready' iv_1 = lv_model_id ).
36: MESSAGE lv_msg TYPE 'S'.
37:
38: ELSEIF lr_ml->is_failed( lv_model_id ) = abap_true.
39:
40: "...creation failed
41: lv_msg = /snp/cn00_cl_string_utils=>text( iv_text = 'Model &1 has failed' iv_1 = lv_model_id ).
42: MESSAGE lv_msg TYPE 'S' DISPLAY LIKE 'E'.
43:
44: ENDIF.
45:
46: CATCH cx_root INTO lr_ex.
47:
48: "output errors
49: lv_msg = lr_ex->get_text( ).
50: PERFORM display_lines USING lv_msg.
51:
52: ENDTRY.
53:
54: ENDFORM.
And now let's break it down into it's individual parts:
Fetch Data into an Internal Table
In my particular case I was fetching the data via a REST Service from the SNP Data Cockpit instance I am using to keep statistics on all executed SNP System Scans. However, you can basically fetch your data that will be used as a data source for your model in any way that you like. Most probably you will be using OpenSQL SELECTs to fetch the data accordingly. Resulting data looks somewhat like this:
Prepare Data
This is the raw data and it's not perfect! The data quality is not quite good and in the shape that it's in. According to this article there are some improvements that I need to do in order to improve its quality.
- Normalizing values (e.g. lower casing, mapping values or clustering values). E.g.
- Combining the database vendor and the major version of the database because those two values only make sense when treated in combination and not individually
- Clustering the database size to 1.5TB chunks as these values can be guessed easier when executing predictions
- Clustering the runtime into exponentially increasing categories (although this may also hurt accuracy...)
- Filling up empty values with reasonable defaults. E.g.
- treating all unknown SAP client types as test clients
- Make values and field names more human readable. This is not necessary for the machine learning algorithms, but it makes for better manual result interpretation
- Removing fields that do not make good features, like
- IDs
- fields that cannot be provided for later predictions, because values cannot be determined easily or intuitively
- Remove records that still do not have good data quality. E.g. missing values in
- database vendors
- SAP system types
- customer industry
- Remove records that are not representative. E.g.
- they refer to scans with exceptionally short runtimes probably due to intentionally limiting the scope
- small database sizes that are probably due to non productive systems
1: FORM prepare_data USING it_data TYPE table CHANGING rr_data TYPE REF TO data.
2: *"--- DATA DEFINITION -------------------------------------------------
3: DATA: lr_q TYPE REF TO /snp/cn01_cl_itab_query.
4:
5: *"--- PROCESSING LOGIC ------------------------------------------------
6: CREATE OBJECT lr_q.
7:
8: "selecting the fields that make good features
9: lr_q->select( iv_field = 'COMP_VERSION' iv_alias = 'SAP_SYSTEM_TYPE' ).
10: lr_q->select( iv_field = 'DATABASE' iv_uses_fields = 'NAME,VERSION' iv_cb_program = sy-repid iv_cb_form = 'ON_VIRTUAL_FIELD' ).
11: lr_q->select( iv_field = 'DATABASE_SIZE' iv_uses_fields = 'DB_USED' iv_cb_program = sy-repid iv_cb_form = 'ON_VIRTUAL_FIELD' ).
12: lr_q->select( iv_field = 'OS' iv_alias = 'OPERATING_SYSTEM' ).
13: lr_q->select( iv_field = 'SAP_CLIENT_TYPE' iv_uses_fields = 'CCCATEGORY' iv_cb_program = sy-repid iv_cb_form = 'ON_VIRTUAL_FIELD' ).
14: lr_q->select( iv_field = 'COMPANY_INDUSTRY1' iv_alias = 'INDUSTRY' ).
15: lr_q->select( iv_field = 'IS_UNICODE' iv_cb_program = sy-repid iv_cb_form = 'ON_VIRTUAL_FIELD' ).
16: lr_q->select( iv_field = 'SCAN_VERSION' ).
17: lr_q->select( iv_field = 'RUNTIME' iv_uses_fields = 'RUNTIME_HOURS' iv_cb_program = sy-repid iv_cb_form = 'ON_VIRTUAL_FIELD' ).
18:
19: "perform the query on the defined internal table
20: lr_q->from( it_data ).
21:
22: "filter records that are not good for results
23: lr_q->filter( iv_field = 'DATABASE' iv_filter = '-' ). "no empty values in the database
24: lr_q->filter( iv_field = 'SAP_SYSTEM_TYPE' iv_filter = '-' ). "no empty values in the SAP System Type
25: lr_q->filter( iv_field = 'INDUSTRY' iv_filter = '-' ). "no empty values in the Industry
26: lr_q->filter( iv_field = 'RUNTIME_MINUTES' iv_filter = '>=10' ). "Minimum of 10 minutes runtime
27: lr_q->filter( iv_field = 'DATABASE_GB_SIZE' iv_filter = '>=50' ). "Minimum of 50 GB database size
28:
29: "sort by runtime
30: lr_q->sort( 'RUNTIME_MINUTES' ).
31:
32: "execute the query
33: rr_data = lr_q->run( ).
34:
35: ENDFORM.
Basically the magic is done using the SNP/CN01_CL_ITAB_QUERY class, which is part of the SNP Transformation Backbone framework. It enables SQL like query capabilities on ABAP internal tables. This includes transforming field values, which is done using callback mechanisms.
1: FORM on_virtual_field USING iv_field is_record TYPE any CHANGING cv_value TYPE any.
2:
3: "...
4:
5: CASE iv_field.
6: WHEN 'DATABASE'.
7:
8: "combine database name and major version to one value
9: mac_get_field 'NAME' lv_database.
10: mac_get_field 'VERSION' lv_database_version.
11: SPLIT lv_database_version AT '.' INTO lv_database_version lv_tmp.
12: CONCATENATE lv_database lv_database_version INTO cv_value SEPARATED BY space.
13:
14: WHEN 'DATABASE_SIZE'.
15:
16: "categorize the database size into 1.5 TB chunks (e.g. "up to 4.5 TB")
17: mac_get_field 'DB_USED' cv_value.
18: lv_p = ( floor( cv_value / 1500 ) + 1 ) * '1.5'. "simple round to full 1.5TB chunks
19: cv_value = /snp/cn00_cl_string_utils=>text( iv_text = 'up to &1 TB' iv_1 = lv_p ).
20: TRANSLATE cv_value USING ',.'. "translate commas to dots to the CSV does not get confused
21:
22: WHEN 'SAP_CLIENT_TYPE'.
23:
24: "fill up the client category type with a default value
25: mac_get_field 'CCCATEGORY' cv_value.
26: IF cv_value IS INITIAL.
27: cv_value = 'T'. "default to (T)est SAP client
28: ENDIF.
29:
30: WHEN 'IS_UNICODE'.
31:
32: "convert the unicode flag into more human readable values
33: IF cv_value = abap_true.
34: cv_value = 'unicode'.
35: ELSE.
36: cv_value = 'non-unicode'.
37: ENDIF.
38:
39: WHEN 'RUNTIME'.
40:
41: "categorize the runtime into human readable chunks
42: mac_get_field 'RUNTIME_HOURS' lv_int.
43: IF lv_int <= 1.
44: cv_value = 'up to 1 hour'.
45: ELSEIF lv_int <= 2.
46: cv_value = 'up to 2 hours'.
47: ELSEIF lv_int <= 3.
48: cv_value = 'up to 3 hours'.
49: ELSEIF lv_int <= 4.
50: cv_value = 'up to 4 hours'.
51: ELSEIF lv_int <= 5.
52: cv_value = 'up to 5 hours'.
53: ELSEIF lv_int <= 6.
54: cv_value = 'up to 6 hours'.
55: ELSEIF lv_int <= 12.
56: cv_value = 'up to 12 hours'.
57: ELSEIF lv_int <= 24.
58: cv_value = 'up to 1 day'.
59: ELSEIF lv_int <= 48.
60: cv_value = 'up to 2 days'.
61: ELSEIF lv_int <= 72.
62: cv_value = 'up to 3 days'.
63: ELSE.
64: cv_value = 'more than 3 days'.
65: ENDIF.
66:
67: ENDCASE.
68:
69: ENDFORM.
After running all those preparations, the data is transformed into a record set that looks like this:
Create a Model
Ok, preparing data for a model is something that the developer has to do for each individual problem he wants to solve. But I guess this is done better if performed in a well known environment. After all this is the whole purpose of the ABAP API. Now we get to the parts that's easy, as creating the model based on the internal table we have prepared so far is fully automated. As a developer you are completely relieved from the following tasks:- Converting the internal table into CSV
- Uploading it into an AWS S3 bucket and assigning the correct priviledges, so it can be used for machine learning
- Creating a data source based on the just uploaded AWS S3 object and providing the input schema (e.g. which fields are category fields, which ones are numeric etc.). As this information can automatically be derived from DDIC information
- Creating a model from the datasource
- Training the model
- Creating an URL Endpoint so the model can be used for predictions as seen in the previous article.
1: FORM create_model USING ir_aws_machine_learning TYPE REF TO /snp/aws00_cl_ml
2: it_table TYPE table
3: CHANGING rv_model_id.
4:
5: rv_model_id = ir_aws_machine_learning->create_model(
6:
7: "...by creating a CSV file from an internal table
8: " and upload it to AWS S3, so it can be used
9: " as a machine learning data source
10: it_table = it_table
11:
12: "...by defining a target field that is used
13: iv_target_field = 'RUNTIME'
14:
15: "...(optional) by defining a title
16: iv_title = 'Model for SNP System Scan Runtimes'
17:
18: "...(optional) to create an endpoint, so the model
19: " can be used for predictions. This defaults to
20: " true, but you may want to switch it off
21:
22: " IV_CREATE_ENDPOINT = ABAP_FALSE
23:
24: "...(optional) by defining fields that should be
25: " treated as text rather than as a category.
26: " By default all character based fields are treated
27: " as categorical fields
28:
29: " IV_TEXT_FIELDS = 'COMMA,SEPARATED,LIST,OF,FIELDNAMES'
30:
31: "...(optional) by defining fields that should be
32: " treated as numerical fields rather than categorical
33: " fields. By detault the type will be derived from the
34: " underlying data type, but for convenience reasons
35: " you may want to use this instead of creating and
36: " filling a completely new structure
37:
38: " IV_NUMERIC_FIELDS = 'COMMA,SEPARATED,LIST,OF,FIELDNAMES'
39:
40: "...(optional) by defining if you want to create the model
41: " synchronously or asynchronously. By default a the
42: " datasource, model, evaluation and endpoint are created
43: " synchronously so that after returning from the method call
44: " you can immediately start with predictions.
45:
46: " IV_WAIT = ABAP_TRUE by default
47: " IV_SHOW_PROGRESS = ABAP_TRUE by default
48: " IV_REFRESH_RATE_IN_SECS = 5 seconds by default
49:
50: ).
51:
52: ENDFORM.
As you see, most stuff is optional. Sane default values are provided that assume synchronously uploading the data, creating the datasource, model, training and endpoint. So you can directly perform predictions afterwards. Creating all of this in an asynchronous fashion is also possible. Just in case you do not rely on performing predictions directly. After all, the whole process takes up 10 to 15 minutes - which is why showing progress becomes important, especially since you do not want to run into time out situations, when doing this in online mode with a GUI connected.
The Result
After all is done, you can perform predictions. Right let's just hop over into AWS machine learning console and see the results:A CSV file was created in an AWS S3 bucket...
...and finally we can inspect the model performance.
Conclusion
This is a big step towards making Machine Learning available to many without the explicit need to cope with vendor specific aspects. However understanding the principles of machine learning, especially in regards to the problems, you can apply it to and what good data quality means for good predictions is a requirement.

0 comments:
Post a Comment